Understanding Gemma 3 Models
Delving into the realm of Gemma 3 Models provides a glimpse into the diverse range of models available and their unique specializations and capabilities.
Variety of Gemma Models
Gemma models offer users a plethora of choices, with a range of sizes, capabilities, and task-specific variations to aid in the creation of tailor-made generative solutions. The Gemma model family encompasses both general-purpose variants and specialized models designed for specific tasks. These models are available in various parameter sizes, catering to different needs concerning capabilities and computational requirements.
Specializations and Capabilities
The Gemma 3 models have undergone rigorous evaluation across multiple benchmarks, demonstrating competitive performance in the realm of AI. For instance, Gemma 3 boasts an impressive Elo score of 1339 in the LMSys Chatbot Arena, securing a position among the top 10 models in terms of performance. Noteworthy strengths of Gemma 3 lie in areas such as reasoning abilities, mathematical skills, factual accuracy, and multimodal capabilities. However, it may exhibit limitations in basic fact recall. Gemma 3's performance stands out among leading closed models like o1-preview, showcasing its prowess in various cognitive domains.
The design architecture of Gemma 3 focuses on multimodality, enabling the processing of both images and text within models containing 4 billion, 12 billion, and 27 billion parameters. Notably, the attention mechanism within Gemma 3 is tailored to operate distinctively for text and image inputs, featuring bi-directional attention for images and causal attention for text tokens. This approach ensures that the model can comprehensively interpret visual inputs, enhancing its ability to understand and generate contextually relevant outputs.
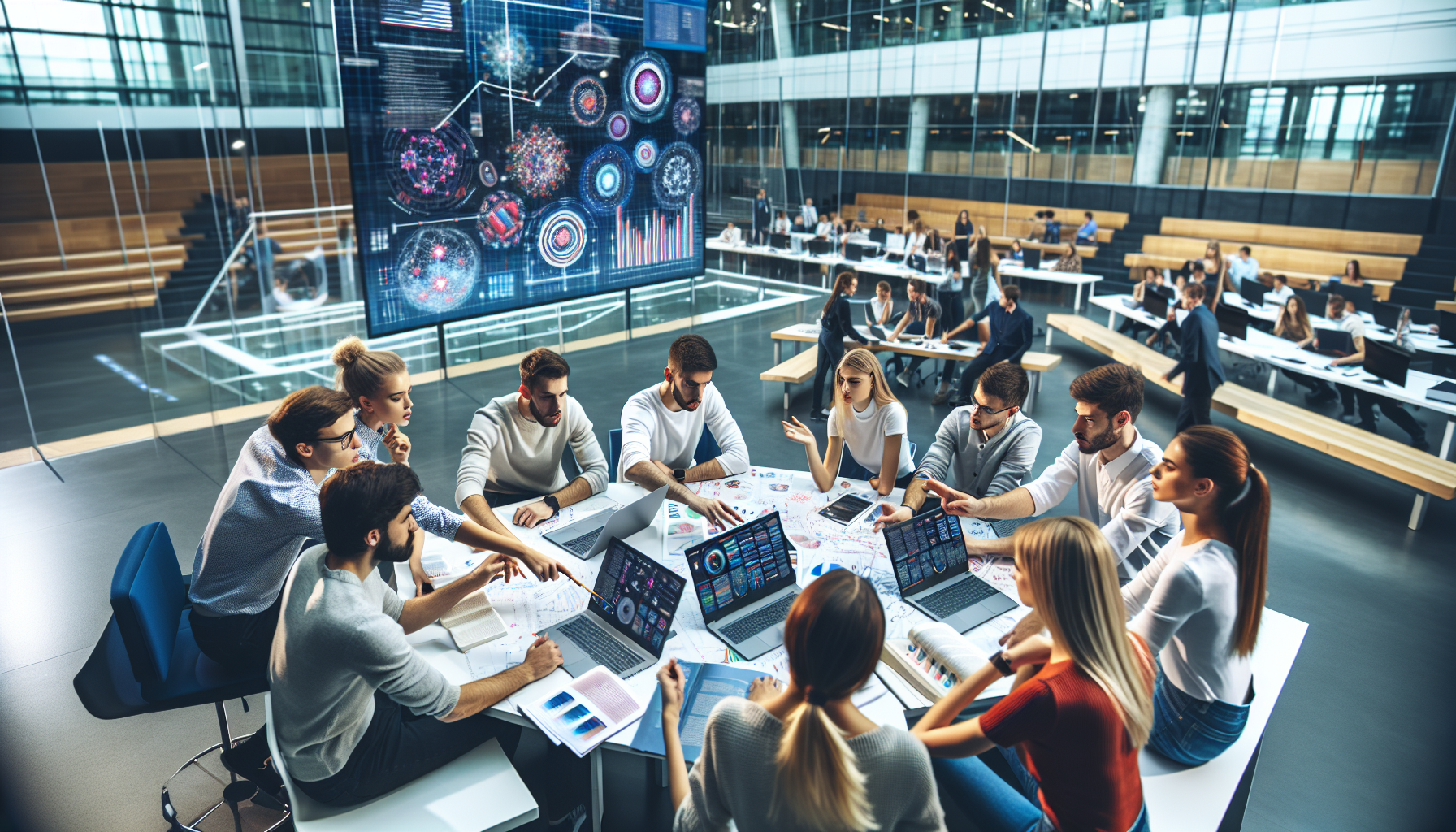
Getting Started with Gemma 3
To effectively utilize Gemma 3 for your AI projects, it's essential to understand the initial steps required to set up the development environment and test the Gemma 3 models.
Setting Up Development Environment
Users can initiate their Gemma 3 journey by setting up a development environment with the necessary components. By downloading the Gemma model and supporting software, users can configure their environment to interact with the model efficiently. This setup enables users to explore the capabilities of Gemma 3 and experiment with its functionalities.
For a more streamlined testing process, users can leverage Google AI Studio, a web application that provides a platform to try out prompts with Gemma 3 without the need for a complex development environment. Within Google AI Studio, users have the flexibility to select different sizes of Gemma models to tailor their testing experience.
Testing Gemma 3 Models
Once the development environment is established, users can proceed to test Gemma 3 models to understand their responses and performance. This testing phase involves prompting the model to evaluate its responses using Python notebooks with preferred machine learning frameworks. Through interactions with the Gemma 3 models, users can assess the accuracy, efficiency, and adaptability of the models to different scenarios and tasks.
Gemma 3 is designed to support on-device usage and is optimized for low-resource devices, with day zero support in mlx-vlm, an open-source library tailored for running vision language models on Apple Silicon devices such as Macs and iPhones. Leveraging Gemma 3's capabilities on a variety of devices ensures accessibility and versatility in deploying AI applications across diverse platforms.
In testing Gemma 3, users will encounter the SigLIP image encoder utilized by the models, which encodes images into tokens for ingestion into the language model. The vision encoder in Gemma 3 processes square images resized to 896x896, implementing an adaptive cropping algorithm known as pan and scan during inference to accommodate non-square aspect ratios and high-resolution images.
To optimize Gemma 3's performance during inference, it is recommended to adhere to specific settings. These settings include a temperature of 1.0, topk of 64, minimump of 0.00 (or 0.01 if default is 0.1), top_p of 0.95, and a repetition penalty of 1.0. By following these guidelines and fine-tuning the configurations, users can enhance the effectiveness and accuracy of Gemma 3 for their AI endeavors.
.png)
Features of Gemma 3
Exploring the capabilities of Gemma 3 unveils a range of cutting-edge features that cater to a diverse array of AI needs. From multimodality to language support and adaptive model sizes, Gemma 3 offers a robust toolkit for AI enthusiasts and professionals alike.
Multimodality and Language Support
Gemma 3 stands out for its seamless integration of both multimodal functionalities and comprehensive language support. This latest iteration of Gemma introduces multimodality with a vision-language input and text output approach, expanding its capabilities to processing a wide range of inputs and generating contextually rich outputs. The platform boasts support for over 140 languages, empowering users to engage with diverse linguistic contexts and bolstering global accessibility.
Incorporating a vision encoder based on SigLIP, Gemma 3 enhances its multimodal prowess by enabling the analysis of images and videos as inputs. This empowers the model to perform tasks such as image analysis, question-answering based on images, image comparison, object identification, and text extraction from images. By seamlessly blending vision and language processing, Gemma 3 offers a comprehensive solution for various AI applications.
Model Sizes and Adaptations
Gemma 3 presents a lineup of models available in four distinct sizes, each tailored to cater to different computational needs and complexities. Ranging from 1 billion to 27 billion parameters, Gemma 3 models offer scalability and versatility, allowing users to select the model size that aligns with their specific requirements. These models come in both base (pre-trained) and fine-tuned versions, ensuring flexibility and adaptability for a wide range of AI tasks.
With a context window extending up to 128k tokens, Gemma 3 models exhibit an impressive capacity to process large volumes of information, enabling in-depth analysis and understanding of complex data sets. Moreover, the models are designed to accommodate both image and text inputs, facilitating seamless multimodal processing and enhancing the overall performance and accuracy of AI tasks. What’s more, supporting over 140 languages, Gemma 3 models embrace linguistic diversity and enable cross-cultural engagement, making them a versatile choice for diverse AI applications.
Gemma 3's adaptive model sizes and multimodal capabilities not only enhance the efficiency and performance of AI tasks but also pave the way for innovative applications across various industries, solidifying its position as a leading AI platform in the realm of multimodal AI processing and adaptive model development.
Maximizing Gemma 3 Performance
To fully optimize the performance of Gemma 3, users can implement specific strategies to fine-tune the models and configure inferences based on their requirements and resources. Tuning Gemma 3 models and setting up inference configurations play a crucial role in enhancing the effectiveness and efficiency of utilizing these advanced AI models.
.png)
Tuning Gemma 3 Models
Tuning Gemma models involves tailoring the model's behavior to suit specific applications by adjusting parameters and training the model on custom datasets. This process requires a dataset of inputs and expected responses with sufficient diversity and scale to guide the model's behavior effectively.
To successfully tune Gemma models, users need significantly more computing and memory resources compared to simply running the model for text generation. It is essential to dedicate the appropriate computational power and time to refine the model's performance and ensure optimal results for the intended tasks.
Inference Configurations
Configuring the inference process for Gemma 3 models is vital for achieving accurate and efficient outcomes. Users can set up inference configurations based on the specific use case and available resources, ensuring seamless integration of Gemma 3 into their applications.
Inference configurations for Gemma 3 models involve defining parameters such as batch size, concurrency, and memory allocation to optimize the model's performance during the inference phase. By fine-tuning these settings, users can enhance the speed and accuracy of model predictions, making the most of Gemma 3's advanced capabilities.
By effectively tuning Gemma 3 models and configuring inferences to align with the desired outcomes and resources, users can leverage the full potential of these multimodal AI models for a wide range of applications. Through careful optimization and customization, Gemma 3 can deliver cutting-edge performance and functionalities, opening up new possibilities in the realm of AI-driven solutions.